Research
Overview
My research bridges artificial intelligence and mathematical modeling frameworks to advance our understanding of immunology, with a particular focus on the interpretability of foundational models in the context of B-cell and T-cell dynamics. I integrate together biological processes spanning multiple scales, such as cell population dynamics, cell-cell interactions, intracellular mechanisms, and protein binding, to construct a realistic in-silico model of the immune response.
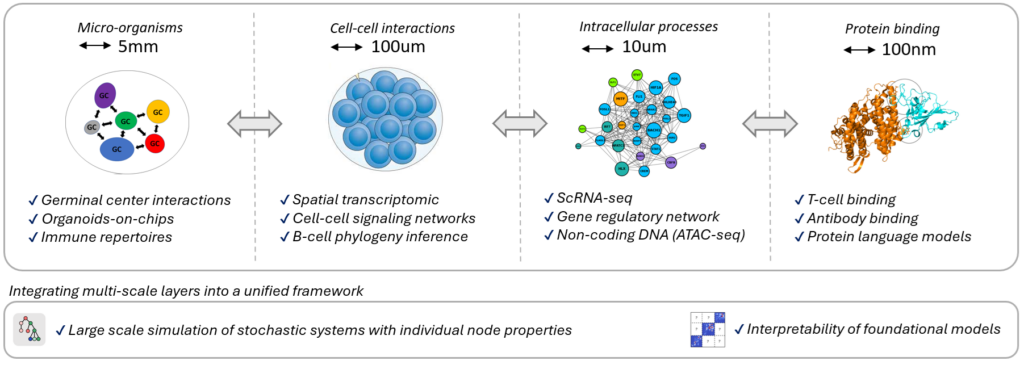
Protein Language Models for Immunology
Department of Biomedical Informatics & Data Science - Yale School of Medicine, United States
With the recent surge in foundational models that have demonstrated the ability to learn and interpret the language of biology at multiple scales (DNA, RNA, transcriptomics), we have seen extraordinary progress across a wide range of biological problems. Protein language models (PLMs) have opened transformative avenues in immunology, addressing challenges once deemed insurmountable. They demonstrate the ability to generalize binding predictions to sequences not represented in training datasets, uncover intricate and non-obvious dependencies between amino acid residues, and design entirely novel protein sequences with optimized therapeutic properties.
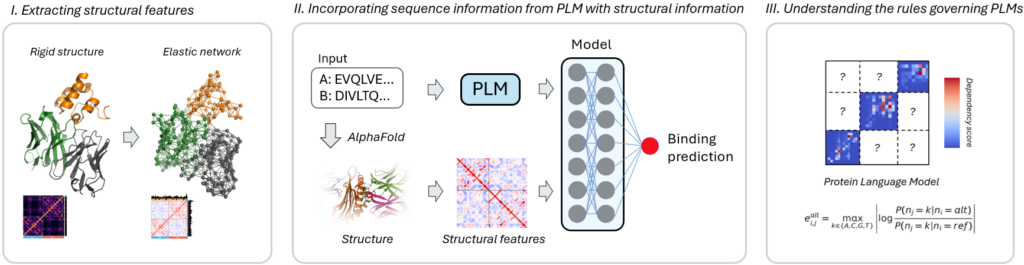
My work investigates how PLMs encode amino acid interdependencies by examining pairwise likelihood maps, capturing the model-inferred probabilities of co-occurrence between amino acid residues, and correlating these with structural features. I investigate how different PLMs represent this information in distinct ways and extend these analyses to capture interdependencies across multiple chains involved in binding antibodies and T-cell complexes, providing insights into the encoding of complex protein interactions.
N. Deutschmann*, A. Pelissier*, A. Weber, S. Gao, J. Bogojeska and M. R. Martinez. Do domain-specific protein language models outperform general models on immunology-related tasks? ImmunoInformatics. (2024)
10.1016/j.immuno.2024.100036
M. Nielsen, A. Eugster, M.F. Jensen, M. Goel, A. Mayer, A. Pelissier, S. Valkiers, M.R. Martínez, B. Piganeeau, V. Greiff, T. Mora, A. Walczak, G. Croce, D. Moreno, D. Gfeller, P. Meysman, J. Barton. Lessons learned from the IMMREP23 TCR-epitope prediction challenge ImmunoInformatics. (2024)
10.1016/j.immuno.2024.100045
A. Weber, A. Pelissier and M. R. Martinez. T-cell receptor binding prediction: A machine learning revolution ImmunoInformatics. (2024)
10.1016/j.immuno.2024.100036
Vascularization of Organoid-on-chips
Life Science Institute - University of British Colombia, Canada
Organoids on chips are three-dimensional tissue models grown in the lab from stem cells, designed to mimic the structure and function of real organs. These organoids are cultivated in a microfluidic platform that provides a controlled environment for their growth and development. Vascularization, or the formation of blood vessel networks within these organoids, is essential for their long-term viability and functionality. It ensures the efficient delivery of oxygen and nutrients and the removal of waste products, which are critical for maintaining healthy and functional tissue structures. Without vascularization, organoids are limited in size and longevity, hindering their potential for accurately modeling human organs and their responses to treatments.
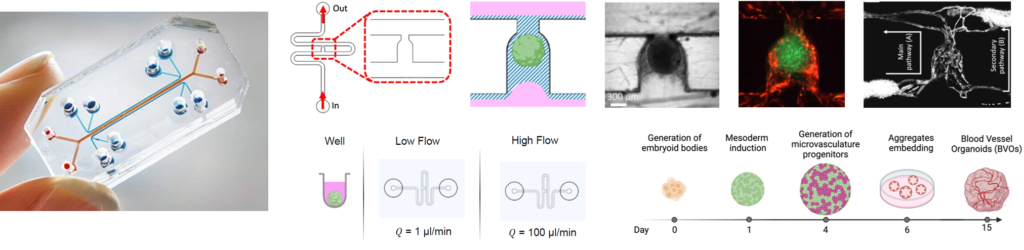
In my research, in collaboration with microfluidic expert and wetlab scientist Clement Quintard, I characterize the benefits of a high-flux environment for the growth of vascularized organoids and the organ-specificity of their vascular networks. By leveraging scRNA analysis to infer cell-cell communication networks, we examine how vascularization influences cellular interactions and the overall functionality of the organoids, providing insights into optimizing their growth and replicating the complexities of real human tissues.
PhD - Multiscale Modeling of the Adaptive Immune System
Laboratory for Computational Systems Biology - IBM Research Zurich, Switzerland
The adaptive immune system consists of specialized cells and processes that eliminate or prevent the growth of foreign pathogens. It can adapt and fight against unseen pathogenes in a remarquably efficient and specific manner, and can also remember past infections with striking precision. In order to build a fast and effective immune response, B-cells duplicate, mutate and go through an accelerated Darwinian selection process. This process occurs in germinal centers (GCs), which thus play a central role in generating an effective immune response against infectious pathogens. Importantly, failures within their tightly regulated environment can lead to the development of autoimmune diseases and cancer. Unfortunatly, there are still many unanswered question about the mechanisms involved in the GC reaction, and it is still the topic of ongoing research.
As GCs are stochastic systems that display a high level of variability even within the same individual, mathematical models are crucial to deepen our understanding of the cellular and molecular processes characterising these complex dynamic systems. Importantly, a realistic model should incorporate together in a hybrid manner the various mechanisms involved in the response, occurring simultaneously at very different scales (e.g. molecular binding, intra-cellular and inter-cellular interactions)
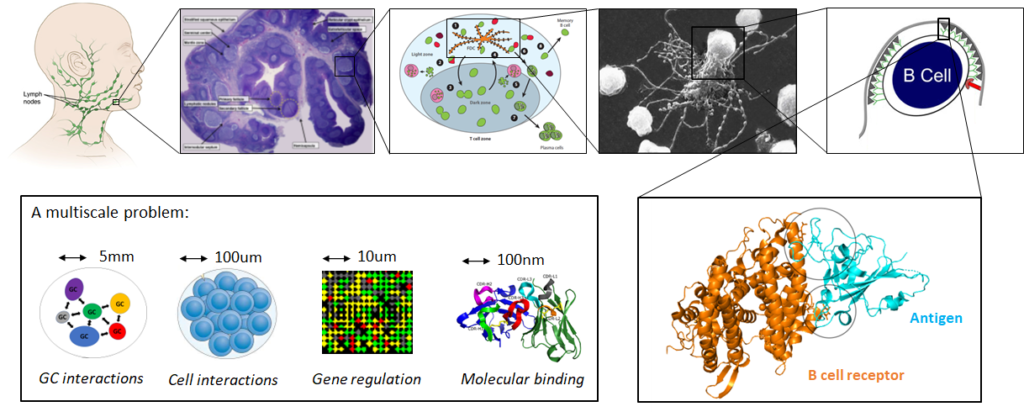
By combining mathematical modeling and artificial intelligence with the analysis of gene expression and RNA sequencing data, my work unravel the complex mechanisms at the heart of our adaptive immune system, which contributes to the understanding of major diseases, such as lymphatic cancer and autoimmune diseases. My research covers B-cell evolution in and accross Germinal Centers, Antigen-Antibody binding predictions, Gene regulatory networks inference, Single cell transcriptomic analysis, and the characterization of non-Markovian dynamics, among others.
A. Pelissier*, T. Laragione*, Carolyn Harris, M. R. Martinez and P. S. Gulko. BACH1 as a key driver in rheumatoid arthritis fibroblast-like synoviocytes identified through gene network analysis. Life Science Alliance 8(1). (2025)
10.26508/lsa.202402808
A. Pelissier, M. Slav, D. Le Bail, N. Beerenwinkel and M. R. Martinez. Practical and scalable simulations of non-Markovian stochastic processes and temporal networks with individual node properties. Nature Computational Science (in revision). (2025)
A. Pelissier, T. Laragione, P. S. Gulko and M. R. Martinez. Cell-specific gene networks and drivers in rheumatoid arthritis synovial tissues. Frontiers in Immunology 15. (2024)
A. Pelissier*, M. Stratigopoulou*, N. Donner, E. Dimitriadis, R. J. Bende, J. E. Guikema, M. R. Martinez, and C. J. van Noesel. Convergent Evolution and B-Cell Recirculation in Germinal Centers in a Human Lymph Node. Life Science Alliance 6(11). (2023)
10.26508/lsa.202301959
A. Pelissier*, S. Luo*, M. Stratigopoulou, J. E. Guikema, and M. R. Martinez. Exploring the impact of clonal definition on B-cell diversity: implications for the analysis of immune repertoires. Frontiers in Immunology 14. (2023)
10.3389/fimmu.2023.1123968
A. Pelissier, Y. Akrout, K. Jahn, J. Kuipers, U. Klein, N. Beerenwinkel and M. Rodríguez Martínez, Computational Model Reveals a Stochastic Mechanism behind Germinal Center Clonal Bursts. Cells, 9(6) (2020).
Stochastic Modeling of the Humoral Immune Response: A Multi-scale Challenge – Supervised by Maria Rodriguez Martinez and Niko Beerenwinkel
PhD Thesis, IBM Research & ETH Zurich, Switzerland (June 2023)
Raman Hyperspectral Imaging for Cancer Diagnosis
Molecule & Life Nonlinear Science Laboratory - Imperial University of Hokkaido, Japan
Raman microscopy is a very promising technique to increase reliability in clinical diagnosis as it provides information on molecular vibrations and crystal structures, while being non-destructive and requiring minimal sample preparation. It allows high spatial resolution and can provide detailed images of individual cells, for which the extraction of chemical and spatial information of their sub-cellular components have the potential to give a more complete understanding of the underlying biological processes as well as better accuracies in clinical diagnosis.
Raman hyperspectral images typically involve huge datasets containing millions of spectra, each with thousands of features, and advanced analytical tools are needed to process the data. A strong knowledge in the biology of human cells along with the physics of Raman spectroscopy is required to account for noise, measurements error, or other factors impacting the measurements. Finally, Raman measurements are typically heavily influenced by experimental conditions, such as light scattering by instruments or unstable auto fluorescence from samples, leading to inconsistency of the Raman intensity spectra over different days of measurement or devices.
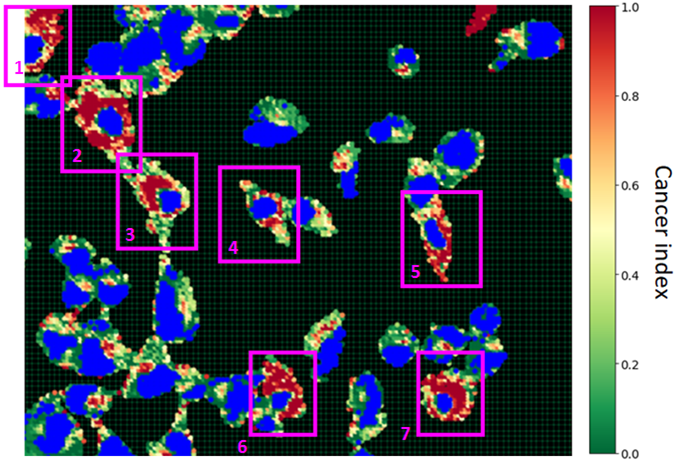
My research involves the development of reliable and robust machine learning and statistical mathematics techniques to analyse spectral imaging data from Raman measurements despite their inconsistencies. The analysis of sub-cellular structures provide increased accuracies in the diagnosis of cancer, and a better understanding of the underlying biological processes as well as possible chemical interpretations behind the detection of malignant cell.
A. Pelissier*, K. Hashimoto*, K. Mochizuki*, J. N. Taylor, JE. Clement, Y. Kumamoto, Y. Harada, K. Fujita and T. Komatsuzaki. Beyond the Nucleus: Cytoplasmic Dominance in Follicular Thyroid Carcinoma Detection Using Single-Cell Raman Imaging Across Multiple Devices. Analytical Chemistry (in revision). (2025).
arxiv.org/abs/1904.05675
J. Taylor, A. Pelissier, K. Mochizuki, K. Hashimoto, Y. Kumamoto, Y. Harada, K. Fujita, T. Bocklitz, and T. Komatsuzaki. Correction for Extrinsic Background in Raman Hyperspectral Images. Analytical Chemistry. (2023)
10.1021/acs.analchem.3c01406
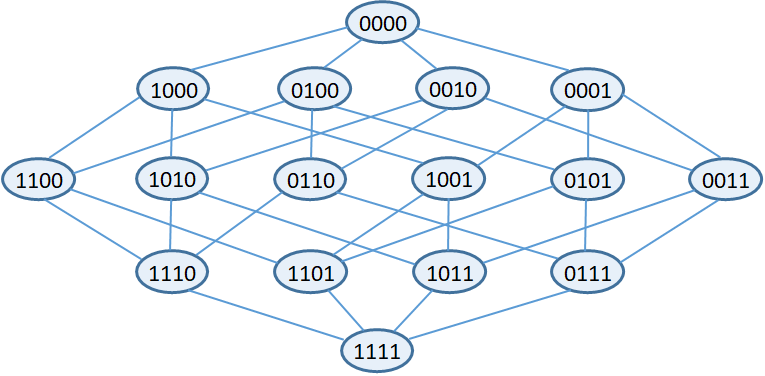
Feature Selection as Reinforcement Learning
Laboratory for Pattern Recognition and Machine Learning - Imperial University of Hokkaido, Japan
Dataset often contains many features that are either redundant or irrelevant, and can thus be removed without incurring much loss of information. Decreasing the number of features have the advantage of reducing over-fitting, simplifying models, and also involve shorter training time, which makes it a key aspect in machine learning. However, the number of subset to consider to find the best combination grows exponentially with the number of features, making the naive method computationally intractable for all but the smallest of feature sets.
In my research, I consider the feature set space as a graph and I use Monte Carlo Search to quickly find an approximated best feature subset in the graph. The crucial trade-off in Monte Carlo Search is between the exploitation of the branch that has the highest expected pay-off and the exploration of the other branch. I explore different possible methods to tackle the exploitation/exploration trade-off during the search, and I aim at finding a policy that provide theoretical guaranty on the best returned feature subset.
A. Pelissier, A. Nakamura, K. Tabata. Feature selection as Monte-Carlo Search in Growing Single Rooted Directed Acyclic Graph by Best Leaf Identification. Proceedings of the 2019 SIAM International Conference on Data Mining, 450-458. (2019).
10.1137/1.9781611975673.51
Feature Selection as Reinforcement Learning Applied to Raman Spectra for Cancer Diagnosis – Supervised by Atsuyoshi Nakamura
M. S. Thesis (2nd year), Imperial University of Hokkaido, Sapporo, Japan (June 2018)
High Harmonic Generation in High Repetition Rate System
Ultrafast Spectroscopy Laboratory - University of British Columbia, BC, Canada
High harmonic generation (HHG) refers to the process of creating vacuum (VUV) or extreme (XUV) ultraviolet light through a nonlinear interaction of an intense laser field with a gas target. It has lead to significant improvements in electron imaging, attosecond physic and photoemission spectroscopy. One of the most charming advantages of HHG sources is their realization on a table-top, making them particularly attractive as an alternative to large-scale facilities, such as synchrotrons or free electron lasers, which only offer restricted user access. However, optimizing the conversion efficiency of the process is challenging because many parameters such as laser intensity, gas pressure or nozzle diameter can be adjusted, and the highly nonlinear phenomenons involved in the harmonic generation process make the choice uncertain.
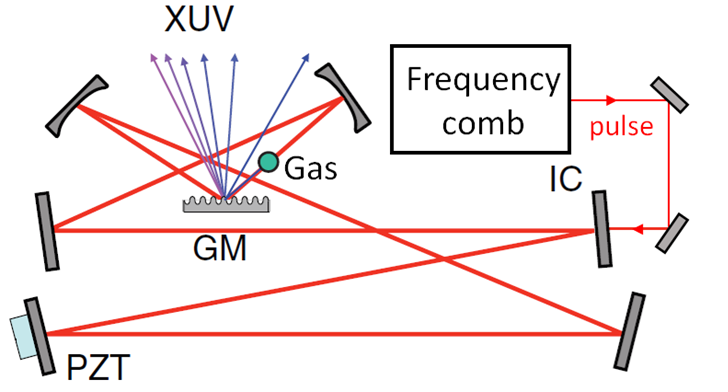
My research consists in analysing the effects of all the parameters involved in high repetition rates HHG systems. The physical phenomenons associated to HHG are various, mainly supersonic gas flow at the nozzle outlet, ions dynamic in the plasma, quantum atomic response of freed electrons, electromagnetic field phase matching, and gas absorption. Numerical simulation results allowed a better understanding of the HHG process, and optimal experimental parameters maximizing the HHG output intensity were proposed.
Study of High Harmonic Generation in High Repetition Rate Systems – Supervised by David Jones
Predoctoral Research Program Thesis, University of British Columbia, Vancouver, Canada (June 2017)
Impact of Piezoelectric Effects on Nanobeam Optical Properties
Advanced Semiconductors for Photonics and Electronic - École Polytechnique Fédérale de Lausanne, Switzerland
A nanobeam is a one dimensional photonic crystal, that consists in our case of a ~100nm holes sequence with growing size in the middle to confine the light. The combination of photonic nanostructures with semiconductor quantum heterostructures has proven successful regarding the achievement of cavity quantum electrodynamics, which may provide a basis for future applications in quantum information processing or schemes for coherent control. To fulfill the conditions for such coupling, a precise tuning of the photonic bandgap and the quantum heterostructure bandgap is needed.
My research focus on tuning the photonic and the heterostructure confinement mode using the piezoelectric properties of III-nitride semiconductor. Due to its piezoelectric nature, the application of an external electric field deforms and bends the nanobeam, resulting in a shifting of its photonic bandgap. The understanding and computation of such process enables fine tuning of the nanobeam optical properties.
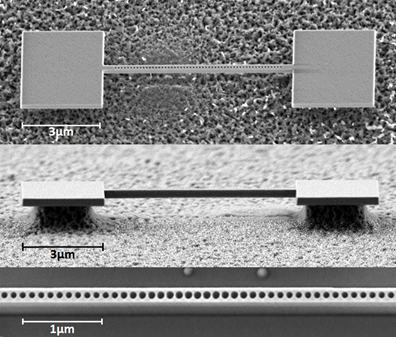
Impact of Piezoelectric Effects on Nitride-III Nanobeam Optical Properties – Supervised by Ian Michael Rousseau, Directed by Raphael Butte and Nicolas Grandjean
M. S. Thesis (1rst year), École Polytechnique Fédérale de Lausanne, Switzerland (July 2016)